Malawi Land Use Change Detection Using Remote Sensing Methods
Environmental degradation is a serious issue in Malawi. Productivity of major crops is significantly below potential yields due in part to Reduced soil fertility caused by unsustainable land use practices are hurting productivity of crops. Environmental data in Malawi have problems of data quality and completeness. Therefore, using satellite images will be a good solution to this. This study aims to identify and quantify the land cover changes in North Malawi from 1994 to 2014 using satellite images and remote sensing techniques.
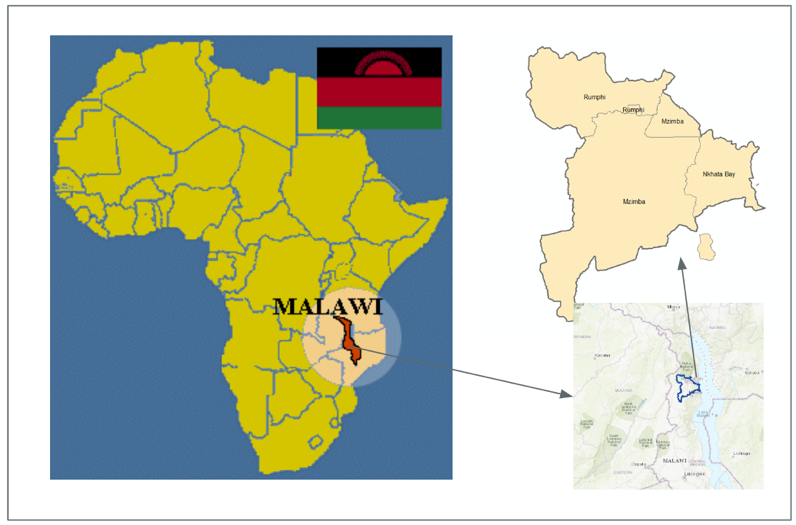
Background
Data source: Two Landsat 8, 30m-resolution images taken on October 1994 and October 2014.
Software: Erdas Imagine and ArcGIS.
Introduction of the Site
Malawi, a landlocked country in southeastern Africa, is defined by its topography of highlands split by the Great Rift Valley and enormous Lake Malawi.
Population: 18.62 million (2017)
The study area in Malawi, which includes parts of 3 districts in Northern Malawi (Mzimba, Nkhata Bay, Rumphi).
Operational Steps
The first step was to geo-reference the images using GCP points (10 GCP points for each image with all of the errors <=0.1). Then, images were cropped to the study boundary. Thirdly, I changed band combination to enhance the images.
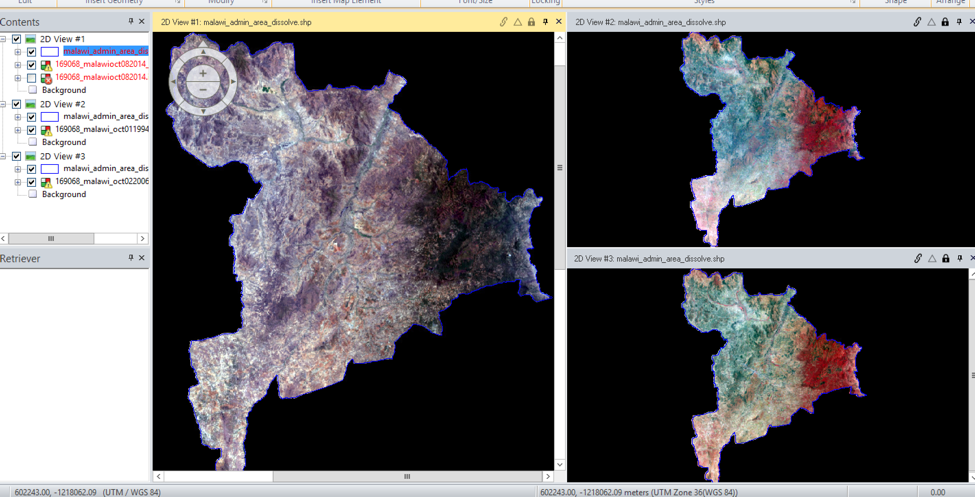
Next, I decided on these six categories as the most common features in the study area:
1: Forest; 2: Shrub; 3: Grass; 4: Crop; 5: Water; 6: Settlement.
Using these categories, I first performed unsupervised classification. I used 36 initial categories and then manually put them into my 6 categories.
Result: Unsupervised Classification: 1994 to 2014.
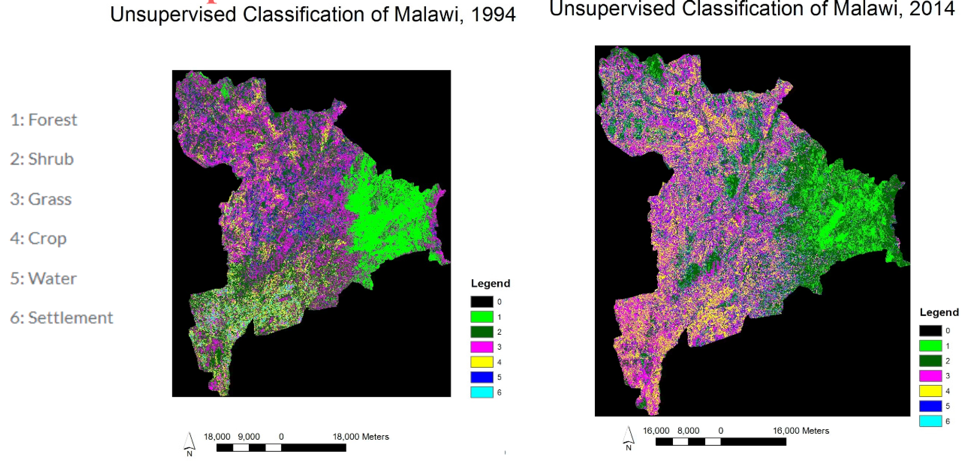
Unsupervised classification of 2014 didn’t identify some settlements in Rumphi Area.
After that, supervised classification was performed using Maximum Likelihood Algorithm. I created a signature file for each image, drew polygons in the image to link to the signature. For each image, I created about 60 signatures. For each categories I created about 10 signatures. Meanwhile, I supervised the distribution of the pixels on the feature space mask, to interactively classify all the signatures. I also made the signatures evenly distribute in the study area.
Result: Supervised Classification: 1994 to 2014.
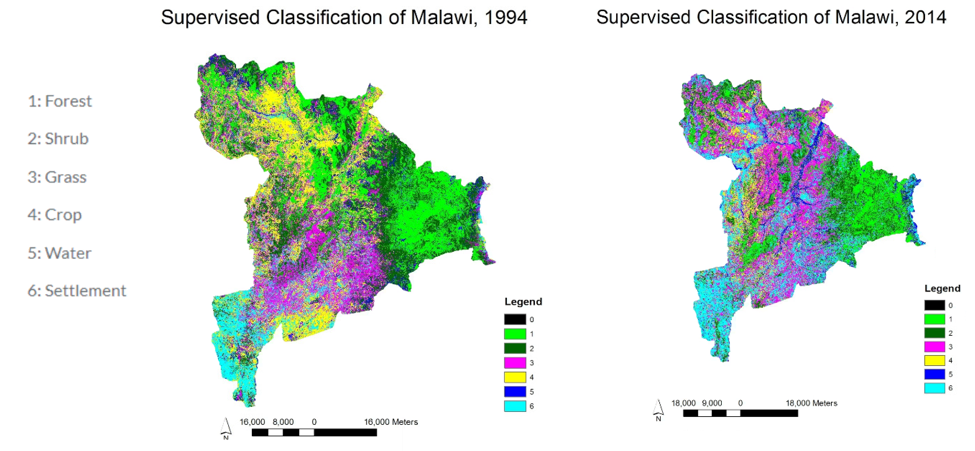
Supervised classification of 2014 also classified more settlements than reality in the south of Mzimba Area.
Then, I decided on the categories of changes. I put all the changes into 7 classes:
I use these 7 classes because I am interested in the changes in vegetation, changes in water, and changes in human settlements.
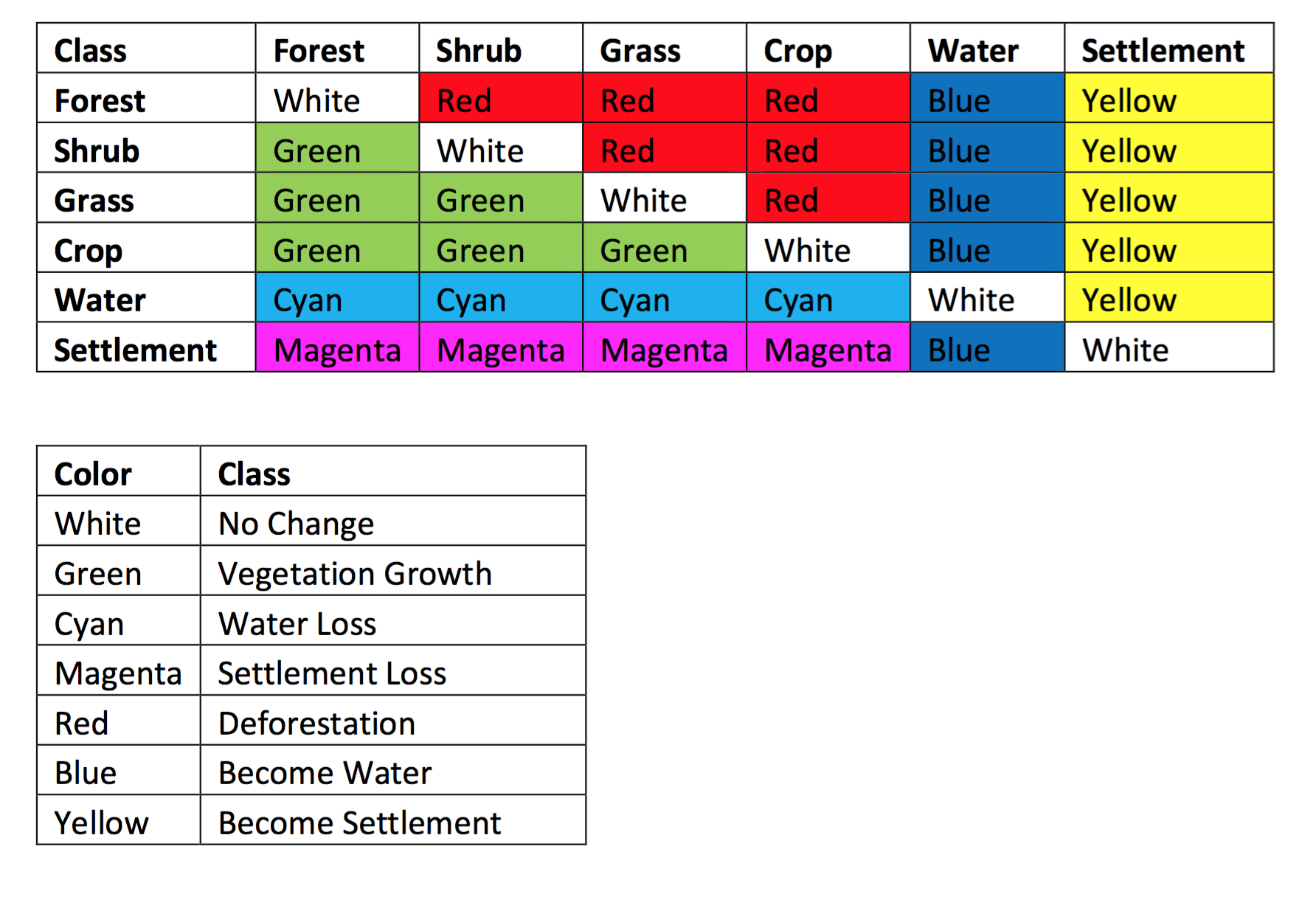
The last step was calculating the number of pixels of those seven changed classes.
Result of change detection using unsupervised classification of 1994 to 2014.
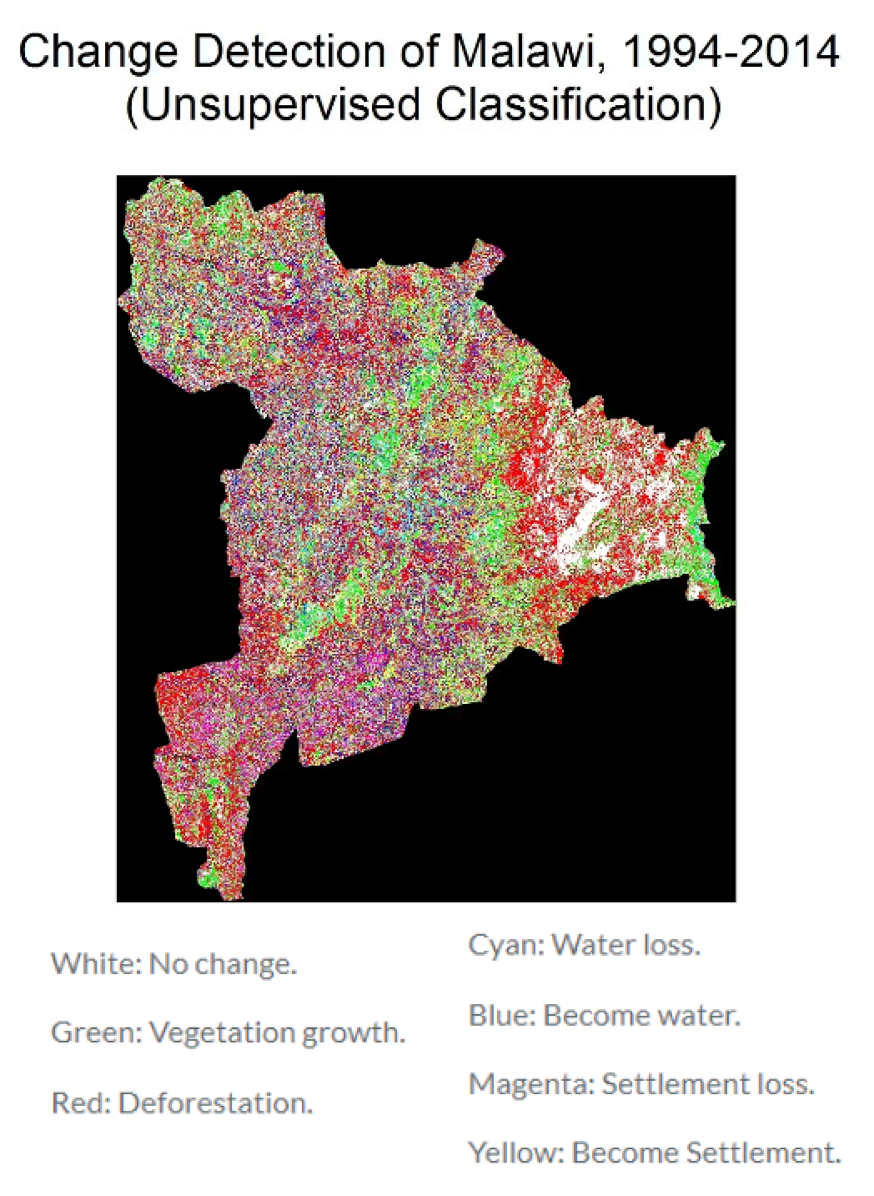
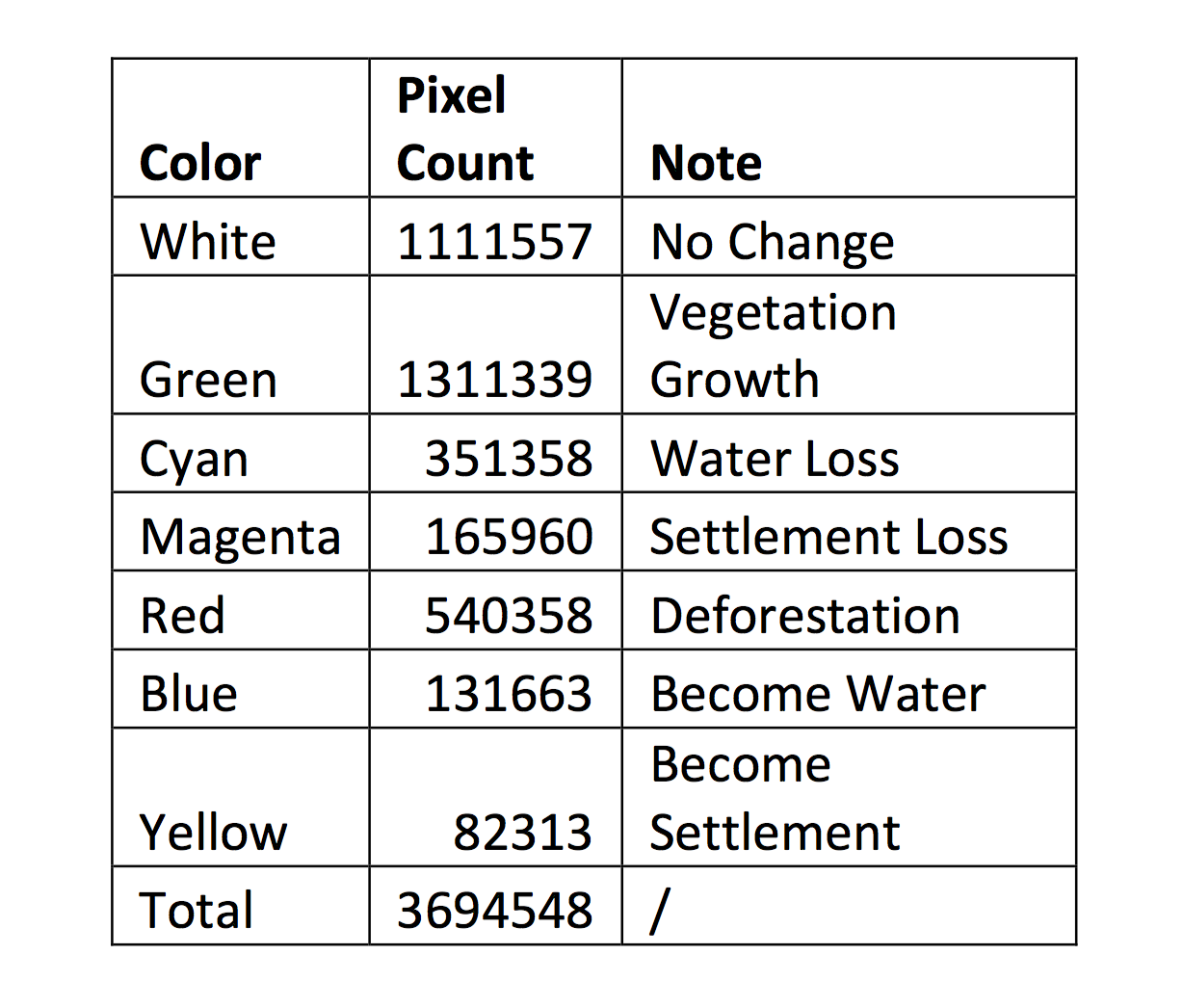
As we can see from the map and the table, most area of lands didn’t change from 1994 to 2014. The most significant change is vegetation growth. Water loss is also very noticeable.
Result of change detection using supervised classification of 1994 to 2014.
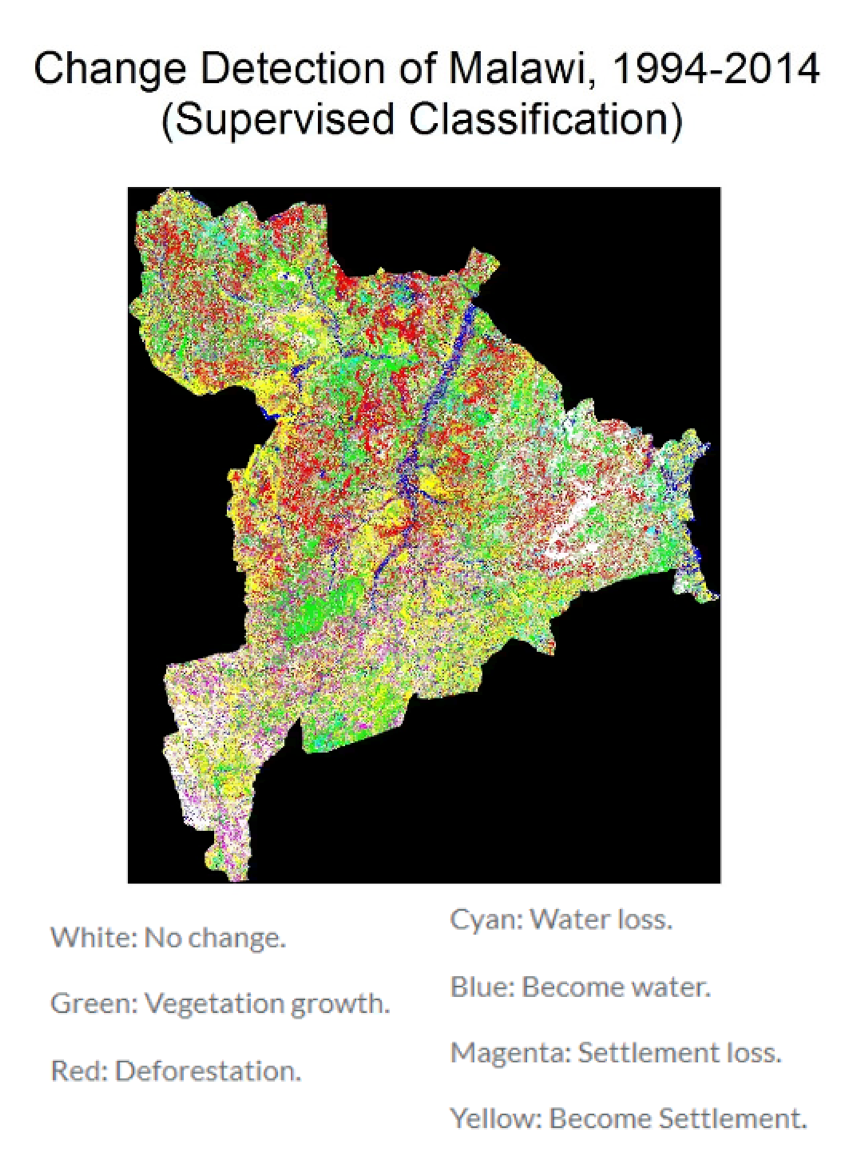
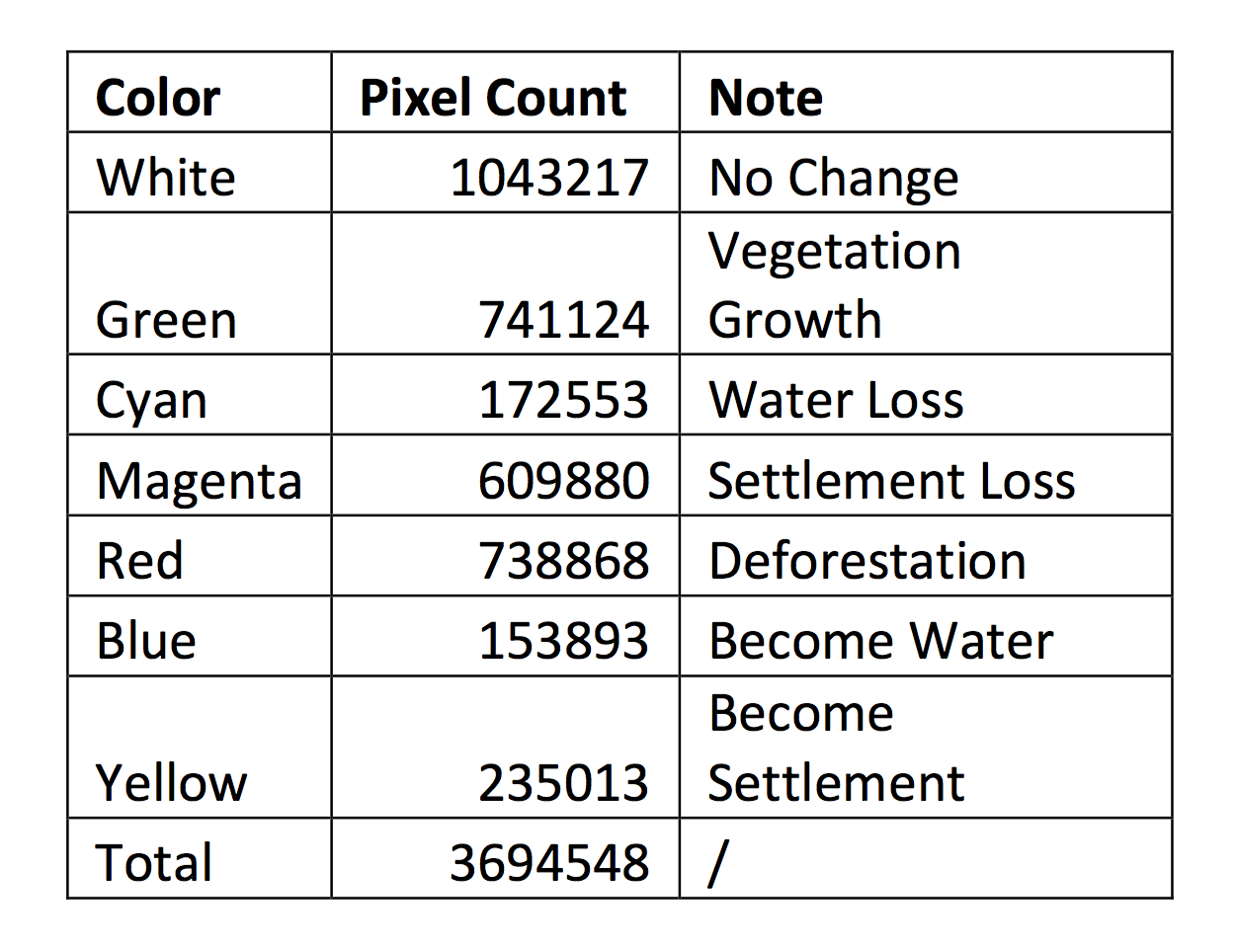
As we can see from the map and the tables, most area of lands didn’t change from 1994 to 2014. Vegetation growth, settlement loss, and deforestation are the three most significant changes.
Discussion
Overall Findings:
• The forest area of the east (Nkhata Bay) didn’t experience obvious change in addition to vegetation growth.
• More crop lands and settlements appeared in the valley area (Mzimba).
• More settlements appeared in the southwest (Mzimba).
• More crop became settlements in the northwest (Rumphi).
• The change detection technique of this area will help crop monitoring, land-use and land-cover (LULC) change monitoring, foreset and urban change monitoring.
Source of Errors:
• Data sources:
Low resolution of Landsat images. (Only 30 meters.)
The roads of the area are not paved. Software tends to classify them into crop/grass.
• Operation:
I am not very familiar with the study area.
Image enhancement can lead to misinterpretation and errors.
Vague information from external reference image, for example: Google Earth.
GCP Selection: errors in photo rectification are not small enough.
Algorithm performance.
• During classification:
Misinterpretation of features.
Errors from the interactive process of selecting signature and masking to feature space. (For supervised classification.)
• During change detection:
Misclassification of zero values and features without signatures.
Algorithm performance.